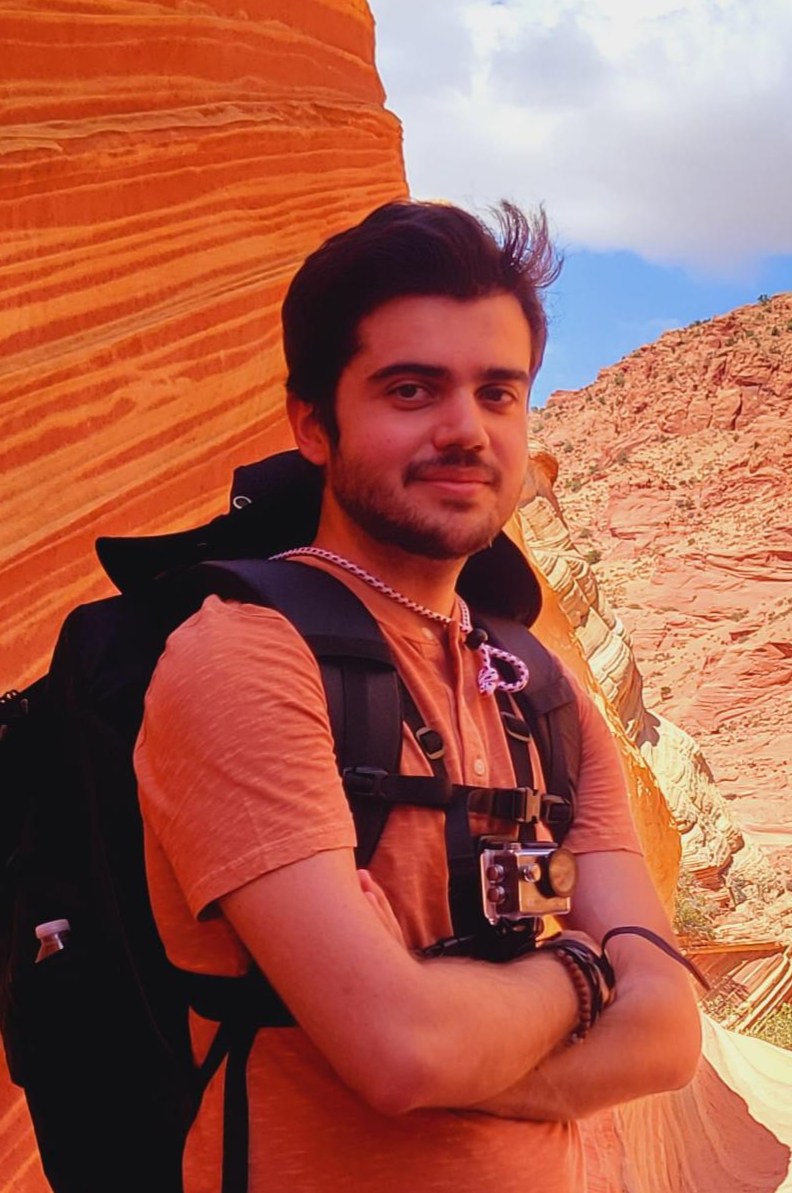
Michael Poli
Staff Scientist at Together.
Computer Science Ph.D. Student at Stanford.
machine learning ∩ systems ∩ signal processing
News:
- Mar, 2024 New research on mechanistic architecture design and scaling laws. Most extensive scaling laws analysis for emerging architectures to date.
- Feb, 2024 We released a new frontier model for biology, Evo! Based on the StripedHyena architecture: Blog.
- Jan, 2024 Podcast on efficient AI architectures for language and beyond: Interconnects.
- Dec, 2023 We released the open-source StripedHyena line of non-Transformer large language models! Outperforms most state-of-the-art Transformers with significant inference throughput improvements: code, HuggingFace, playground.
Research:
My research is at the intersection of machine learning, systems and signal processing:
- Architectures for long context and new applications
T1, Hyena and HyenaDNA, StripedHyena, Evo; - Mechanistic intepretability and design for AI models
Zoology, Mechanistic Architecture Design; - Hybridization of numerical methods and learning
Hypersolvers, Differentiable Multiple Shooting; - Efficient inference and distributed training systems at scale
LaughingHyena;
Short bio:
I am a Staff Scientist at Together and a Ph.D. student in Computer Science at Stanford University. I am grateful to a long list of brilliant researchers and friends that have advised me through the years and (inexplicably) believe in my work:
Stefano Ermon, Chris Ré, Eric Horvitz, Bryan Wilder,
Seong Joon Oh, Animesh Garg, Ilija Ilievski, Jinkyoo Park, among others.
I am originally from Bologna, Italy, and I have had the wonderful opportunity to spend 5 fun years in Asia (China and South Korea).
My Chinese name is 宁致远.